The College of Education and Professional Studies is committed to creating and sustaining an inclusive environment where every student's unique identity is valued and respected, and in which students feel safe to explore their intellectual, interpersonal and professional development in order to serve competently in a multicultural world.
The risk of engaging in bias is always present and requires constant vigilance. Faculty, staff and administrators of the College of Education and Professional Studies are dedicated to making an active, conscious, and intentional effort to provide educational experiences, conduct research, and provide community service in a manner which furthers our collective capacity for civil discourse, which actively combats racism, sexism and other forms of bigotry, and which reflects an appreciation for diverse values and cultures of all people.
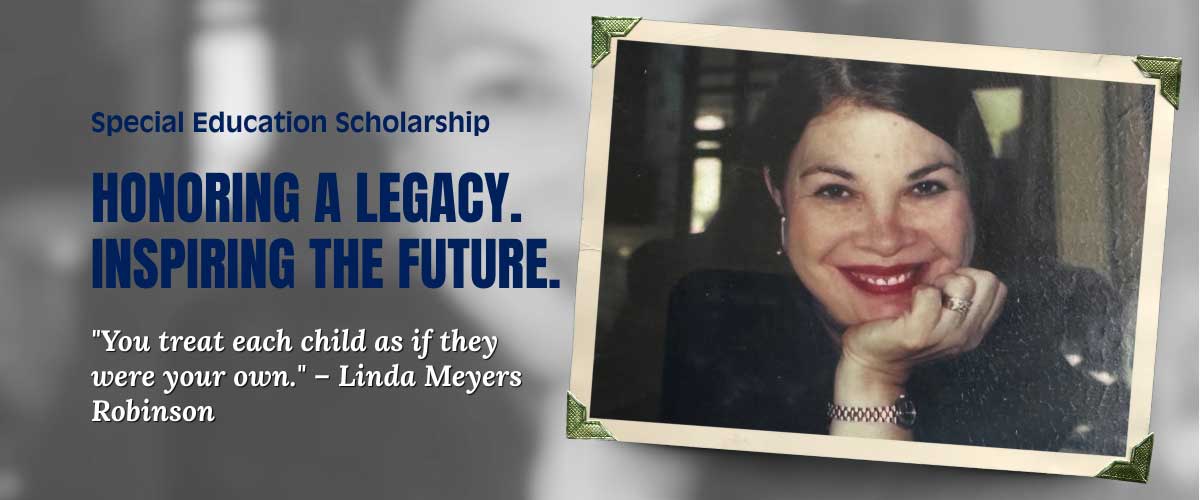
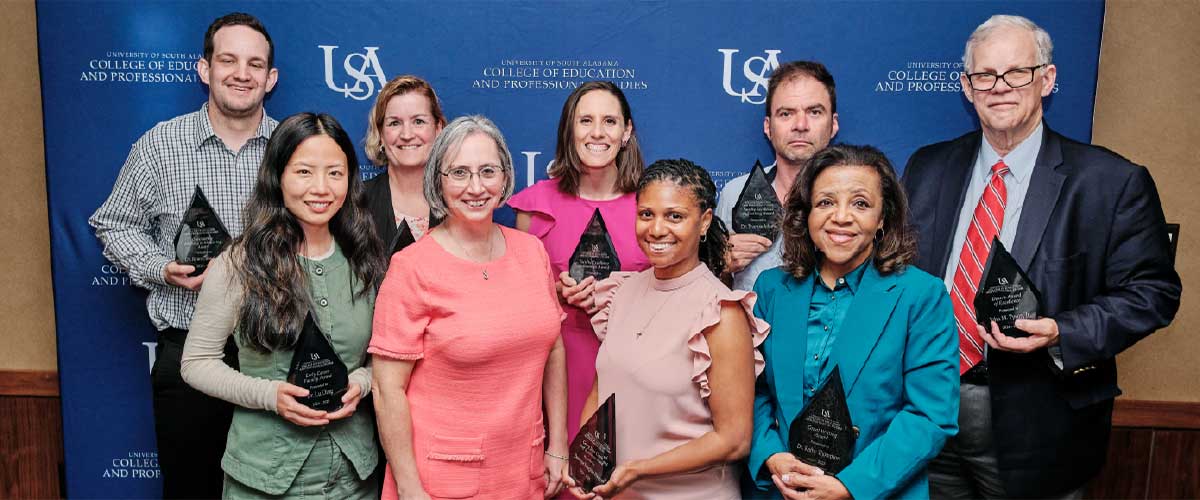
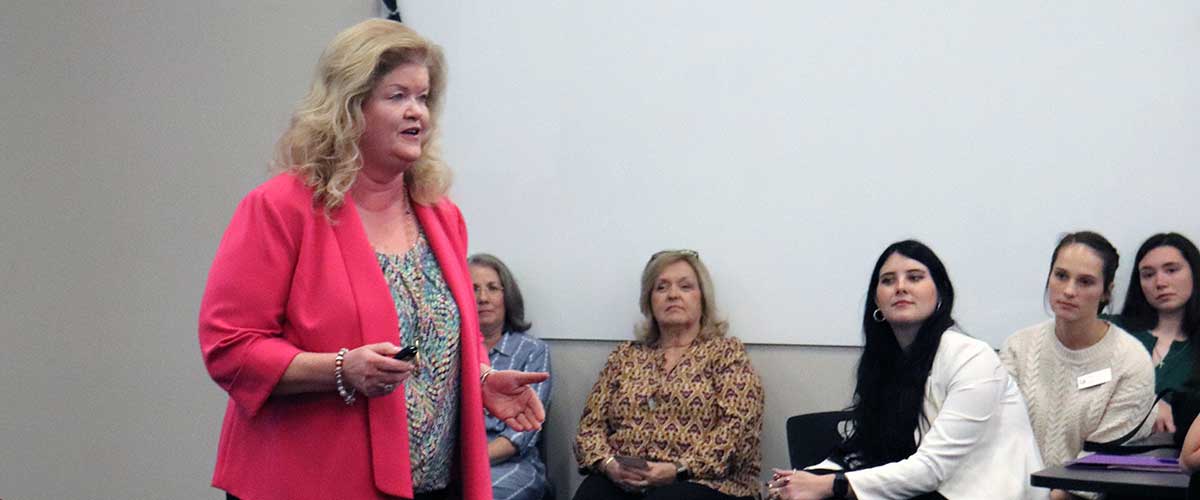